The Digital Imperative
In today’s fast-paced digital age, every company must prioritize becoming a data-centric entity or risk obsolescence. Yet, an alarming statistic from an MIT Survey reveals that a mere 13% of organizations are truly proficient in executing their data strategies.
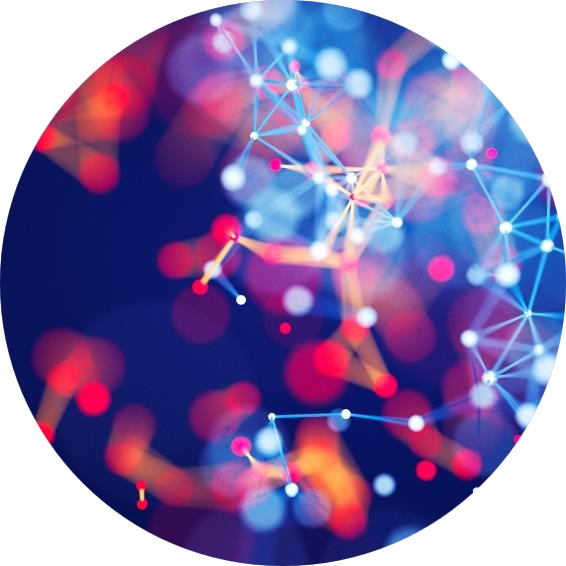
Empowering Organizations for the AI Revolution
While the true potential of AI is vast, it fully unfolds only when organizations effectively balance the initial development costs with the considerable business advantages of broad AI implementation. Let us guide your journey to achieve these transformative gains.
We assist organizations in reimagining their structures, harnessing the unparalleled strength of data, networks, and artificial intelligence. Let’s shape the future together.
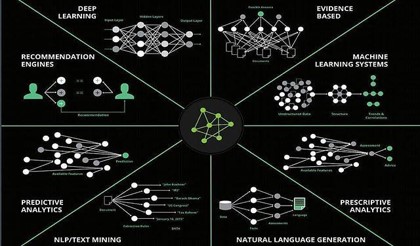
One Strategy
Architectural Clarity
Agile, Product-Focused Organization
Capability Foundation
Clear, Multi-disciplinary Governance
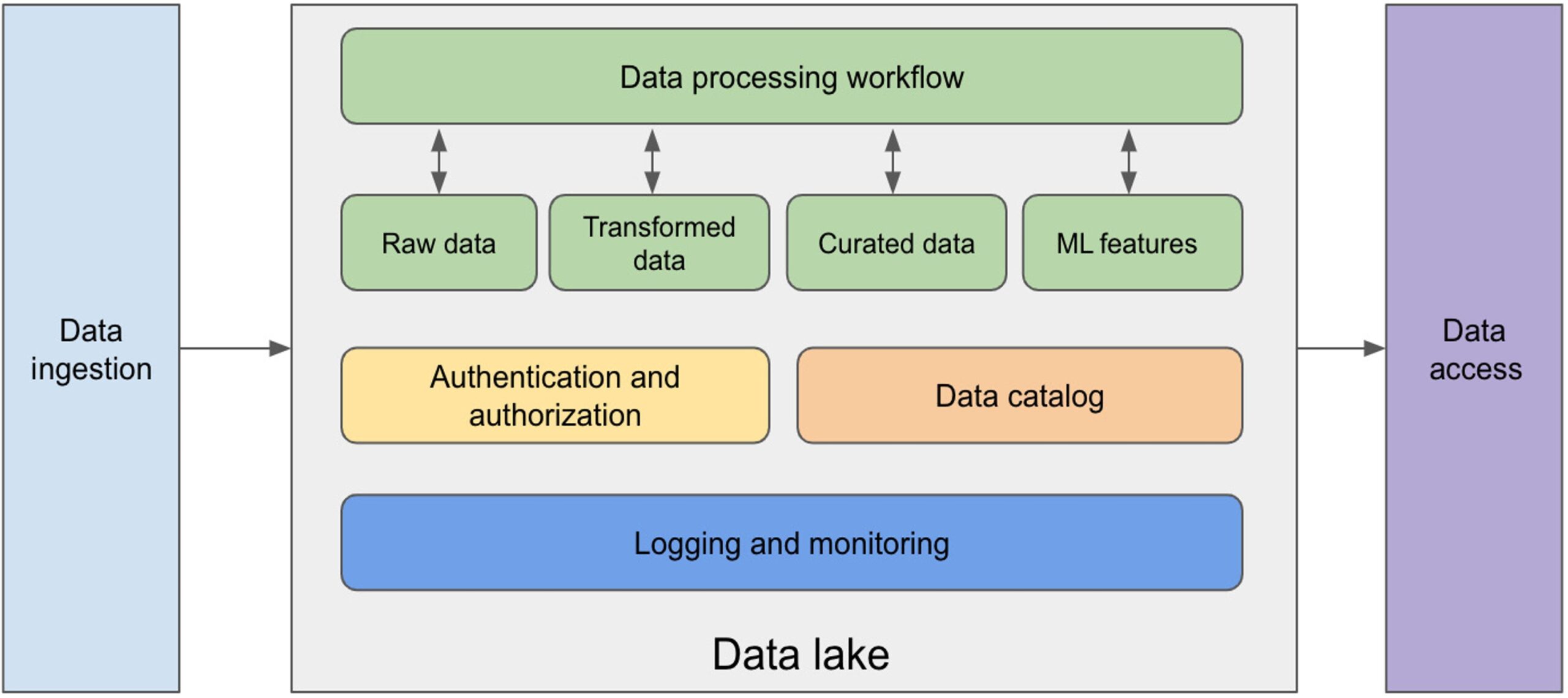
How Do you Build High Performance AI Organization?
- Effective enterprise data strategy, with measurable business impact across multiple business units
- Well-Architected machine learning lifecycle
- Production-grade operations and deployment
- Support for continuous integration (CI), continuous training (CT), and continuous deployment (CD)
- MLOps support
- Support for different languages and ML frameworks
- Integration with other third-party systems and software
- Authentication and authorization
- Artifacts management
The core requirements for enterprise ML platform design and implementation.
- Enterprise ML architecture pattern
- Adopting ML Operations (MLOps) for an ML workflow
- Governance and security
- ML platform poses many unique challenges, such as integrating with the data platform and high-performance computing infrastructure for large-scale model training.
- Support for the end-to-end ML life cycle:
- To enable production-grade operations and deployment, an enterprise ML platform also needs to have architecture components for large-scale model training, model management, feature management, and model hosting with high availability and scalability.
- Support for continuous integration (CI), continuous training (CT), and continuous deployment (CD).
Well-Architected machine learning lifecycle
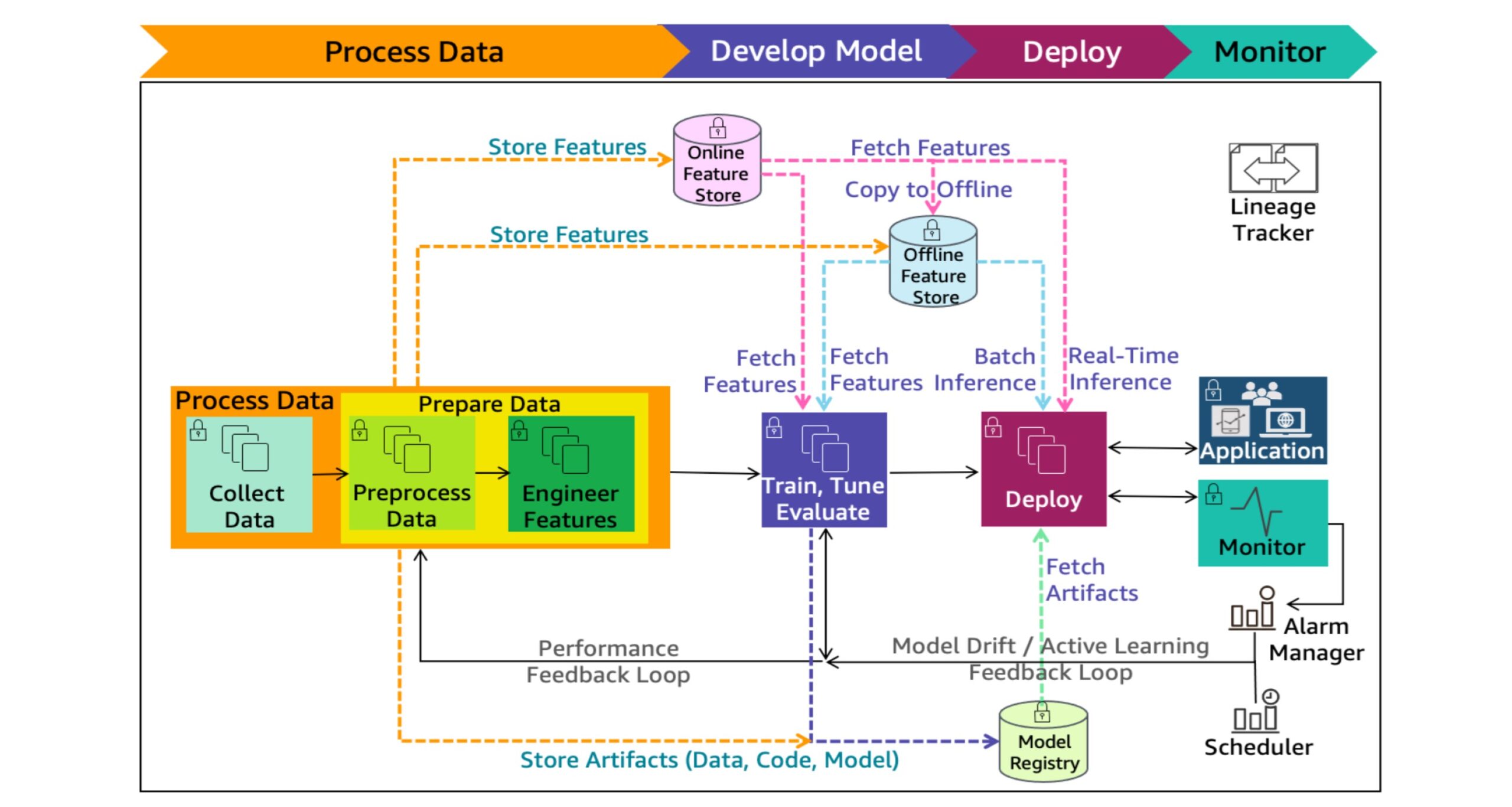
Source: AWS-Whitepaper-Machine Learning Lens
Production-Grade Operations And Deployment
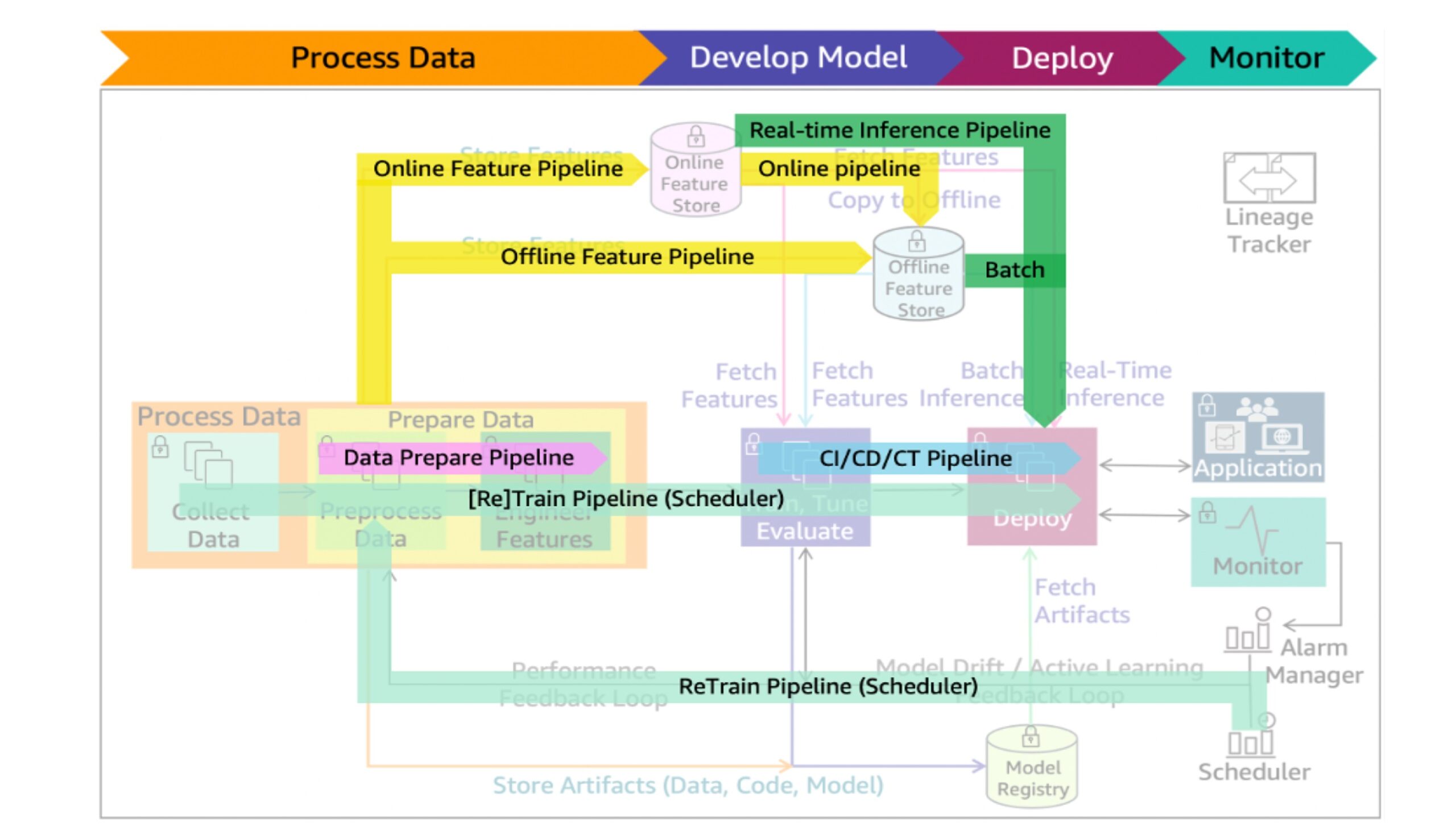